Information and regulation at the origins of life
An ongoing debate in artificial life involves the definition and characterisation of living systems. This definition is often taken for granted in some fields, listing either a set of functions (e.g. reproduction, self-maintenance, metabolism) or a set of properties (e.g. dna) that attempt to fully describe living systems. It is however common nowadays to question most of these attempts, finding possible counterexamples to these arguments (e.g. mules don’t reproduce and viruses, if alive at all, do not contain any DNA), and showing how elusive the definition of life really is. Recent efforts in addressing the origins of life propose to include information theory in order to describe the blurry line between non-living and living architectures.
My work here at ELSI is largely based on this last idea, with a strong drive to include concepts derived from dynamical systems and control theory alongside information theoretical measures. Control theory is the study of regulatory processes mainly deployed in engineering systems, the simplest examples being a thermostat, built for instance to regulate the temperature inside a building, or a cruise controller on a car, maintaining a constant speed despite changes in the environment like wind or the slope of a road. Biological systems show an extraordinary ability to regulate their internal states, from temperature to pH, to different chemical levels. These processes are usually addressed under the umbrella term “homeostasis”, representing the ability of living systems to finely tune conditions for their persistence. (“Homeorhesis” might be more correct in this context, with the former referring to static equilibria and the latter to stable trajectories. For simplicity we will however use the perhaps more familiar term, homeostasis.)
Is there a way to use this remarkable and in some ways maybe unique adaptation to formally characterise the origins of life? Homeostasis is most definitely a requirement for living systems but is it a sufficient condition? Probably not. Replication and other crucial functions of biological systems are, in my opinion, not easily described in terms of regulation alone. Artificial systems can also clearly be built to show some similar properties (e.g. the thermostat mentioned previously). I believe it is however vital to consider its role at the origins of life, as perhaps suggested in the formulation of autopoiesis and related theories.
In order to define homeostasis more rigorously, I explicitly refer to frameworks of control theory and one of their most influential applications to the study of natural sciences in the last century, cybernetics. In particular, a few key results: the “law of requisite variety” then included into the “good regulator theorem” (GRT) and the “internal model principle” (IMP). All of these results seem to point at a common concept for control and homeostasis: regulation implies the presence of a predictive model within the system being regulated. In other words, to maintain certain properties within bounds (i.e. to be a “good regulator”), a system must be able to generate an output (control signal) capable of counteracting the effects of the input disturbances that may affect the system itself. For instance, let’s consider a thermostat trying to regulate the temperature of a room to be around 20°C. If the temperature is 12°, the thermostat must be able to increase the temperature by 8°, if it can’t then it won’t be a good regulator for this system. While this last statement may sound trivially true, it really isn’t since it allows us to say that predictive (or generative) models are present in a system of interest (e.g. one could write down a model of a good thermostat with the necessary information to tune the temperature).
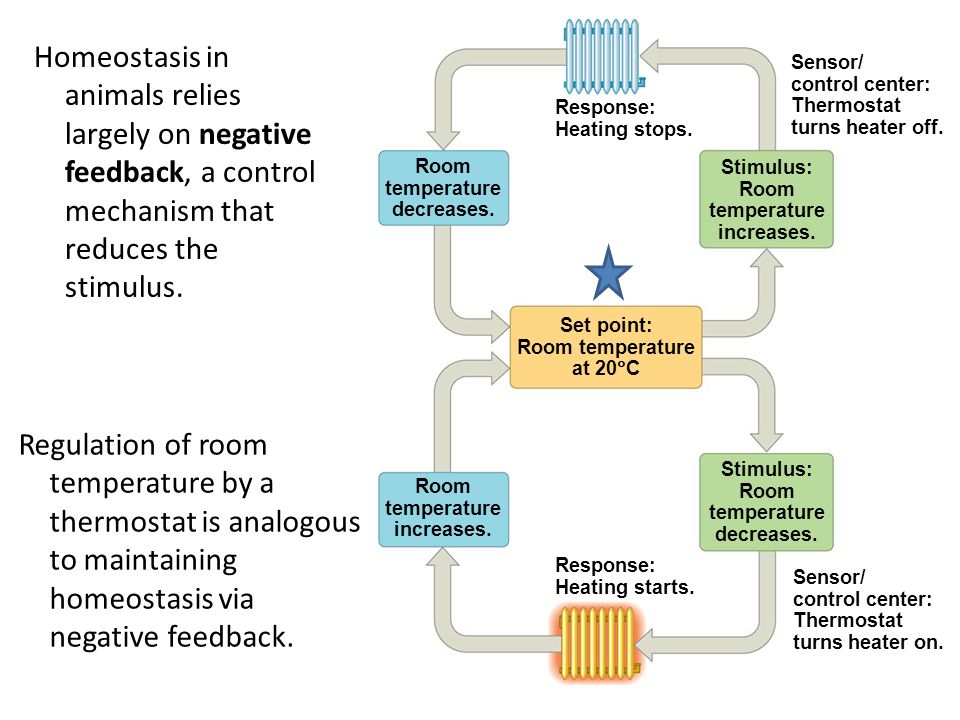
Living systems could metaphorically be seen as very complicated thermostats. They respond to most disturbances avoiding decay, they regulate their temperature alongside several other variables including pH, oxygen intake and various chemical levels. Models of the origins of life should, in my opinion, be able to characterise the abundance of regulatory mechanisms in living systems starting from simpler chemical reactions. Here at EON/ELSI I began investigating models of reaction-diffusion systems. These models try to capture the spatial and temporal changes in concentration of one or more interacting chemicals. The one I focused on, the Grey-Scott system, represents one of the most studied autocatalytic models (an autocatalytic model is one where the product of a chemical reaction is also a catalyst for the same reaction). This model has been previously proposed as a testing ground for theories of the origins of life, as shown in work by NathanielVirgo (now here at EON/ELSI) and colleagues.
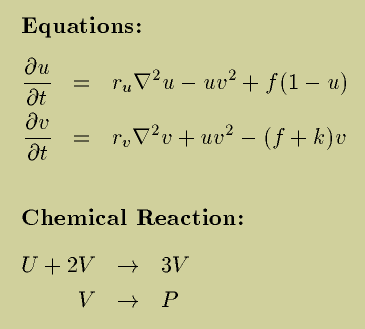
The Grey-Scott system shows a wide variety of patterns emerging through the reaction of as few as two chemicals. Different parameters in the model also allow for different behaviours to emerge, moving patterns as well as ones that don’t move, or blobs that divide in a mitosis-like fashion (for an example refer to this video). In my simulations I focused on two specific patterns, a moving one commonly addressed as “u-skate” given its u-shape and its movements on a straight line if left in isolation, and a stable one also known as a type of “soliton” (non-moving patterns that don’t divide/replicate). Work in this area focuses on the emergence of complex behaviour via the interactions of several patterns, my focus here is however on the analysis of the properties of a single one. For simplicity then, I set up the initial conditions (i.e. dropping a specific quantity of a chemical) necessary for the formation of only one shape per simulation. The goal of these simulations was to identify significant changes in some information quantities between the pattern of interest and its environment. Part of my intentions was also to avoid pre-specifying conditions and rules in order to recognise the pattern itself, looking for ways in which information measures alone could define whether a shape is formed and whether information is stored within it, in a meaningful way. During this study I focused on two questions:
- Can one of these patterns show better predictions of its future states, if compared to its “environment” (i.e. chemicals not forming patterns)? Is it a relevant way of describing the formation of complex structures that could lead to life?
- Can one of these patterns be shown to encode information from its environment? Is there a flow of information from the environment to the pattern showing how information is aggregated in the pattern itself?
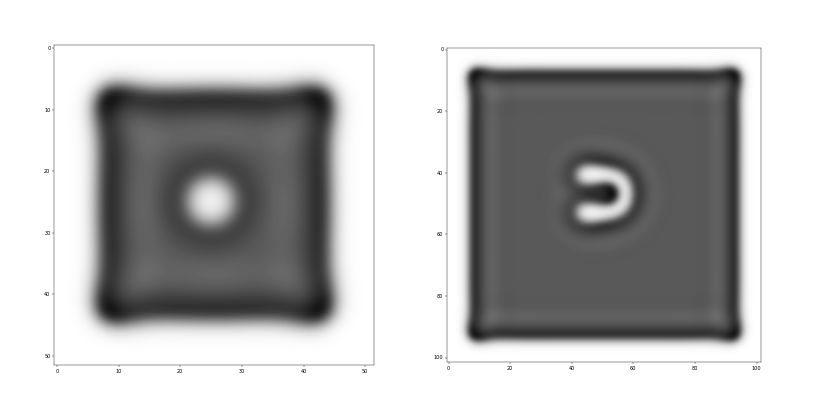
For the analysis, I focused on Predictive Information (PI) and Transfer Entropy (TE) measures. The former was used to investigate if a blob’s ability to predict its future states is qualitatively different when compared to its surroundings or in other words, is self-prediction a good indicator for the emergence of complex structures, maybe life? The latter was used to see whether there is an emergence of directed information exchange between a blob and its environment or in other words, is there a relevant information flow emerging when chemicals organise in more robust structures?
Preliminary results show that PI appears to correlate well with the dynamics of the patterns’ formation, indicating perhaps how information is stored in the pattern itself during its formation. In the case of a soliton over a very long simulation time however, PI shows no difference between solitons and their environments, suggesting that when the chemical system has reached a stable enough state, this quantity is not meaningfully capturing differences between a stable blob and its surroundings. In the case of the u-skate, I tracked the movement of the shape(thus dropping momentarily one of my goals, the automatic recognition of patterns from information measures alone) and measured PI in a moving framework in an attempt to capture the dynamics of this pattern. The results are the moment being analysed, with waves of PI that seem to propagate from the pattern at its formation (probably due to its movement, generated by changes in chemical gradients around the shape). In the long run, for the u-skate too we can see how PI seems not to capture differences between the pattern and the surrounding, with similar levels of PI for different parts of the systems. In both the soliton and the u-skate, the most promising results at the moment seem to emerge from a perturbation analysis that I started recently, with small amounts of chemicals dropped in several areas of the grid (including on the shape itself) at a quite high frequency. In this case, preliminary evidence might suggest how the shapes seem to maintain high PI if compared to the environment in spite of perturbations. My speculation is at the moment that PI might be capturing the robustness to perturbations of the shape, the patterns are more robust and therefore better at predicting their future states when compared to chemicals not organised in patterns.
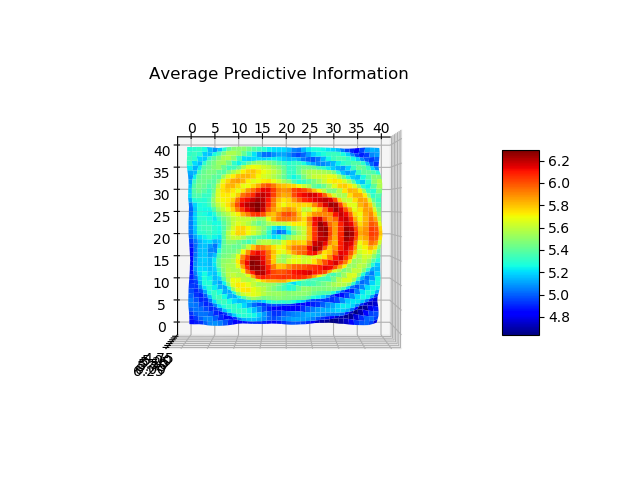
The analysis using Transfer Entropy is still in the workings, with issues mostly due to the fact that it is not computationally feasible to measure TE between all possible combinations of time series even on a discretised grid. We are the moment considering ways to coarse-grain the system if a meaningful way.
To summarise my work (mostly in progress):
- How can we define the emergence of life from chemical systems?
- Can information theory in conjunction with control theory be used to quantify and explain information contents in simple chemical systems that are relevant for the origins of life?
- What are (if any) the relevant informational features of a living system?
In attempt to answer this questions, I set up some simulations with a model of reaction-diffusion equations of two chemicals, the Gray-Scott system. This system is known to show the emergence of many different patterns with quite diverse behaviour and has been suggested before to be a possible test ground for theories of the origins of life (1.).
One of the processes that I would consider general to living systems is homeostasis, the ability to maintain some quantities within boundaries (e.g. temperature, oxygen level). In control theory it is well known that regulation processes (like homeostasis) require the presence of an internal (generative or predictive) model storing information about environmental disturbances affecting the system (2.). In my first attempts to investigate this idea, I focused on two information measures that might help quantifying information in simple patterns of the Gray-Scott system that might relate to the presence of such predictive model. The two measures are Predictive Information (i.e. how much the past of a variable tells you about its own future) and Transfer Entropy (i.e. how much the past of a variable tells you about another variable’s future). The results are still very preliminary, but there is some small hint suggesting that information measures like PI might correlate to a general concept of robustness to perturbations that I believe to be fundamental for living systems.
About the author: Manuel Baltieri
Manuel is a PhD candidate at the University of Sussex, Brighton, UK. His research interests include questions regarding the relationship between information and control theory, biological and physical systems. His research project focuses at the moment on theories of Bayesian inference applied to biology and neuroscience, such as the active inference and predictive coding, and their connections to embodied theories of cognition. In his work, Manuel uses a combination of analytical and computational tools derived from probability/information theory, control theory and dynamical systems theory.
Twitter: @manuelbaltieri